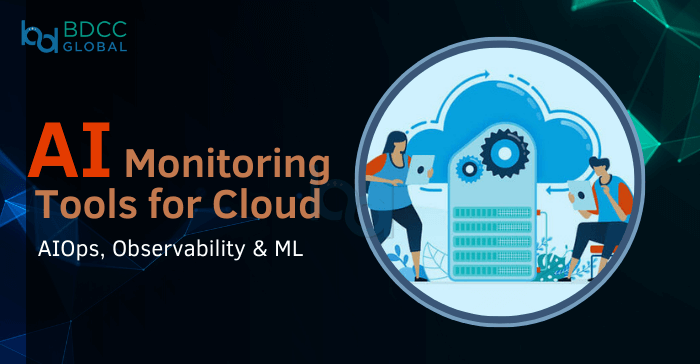
Cloud infrastructure is the backbone of today’s digital enterprises. But as systems become more distributed, dynamic, and complex, traditional monitoring approaches are no longer enough. This is where AI-powered monitoring tools for cloud infrastructure are stepping in; changing the game with intelligent automation, real-time insights, and predictive capabilities.
Whether you’re a business leader trying to optimize costs or a developer ensuring app performance, understanding how AI in cloud infrastructure works can unlock serious operational advantages.
Why Traditional Monitoring Falls Short
Conventional monitoring tools were designed for static environments. They rely on preset rules and thresholds, generating alerts when these are breached. But cloud-native applications are dynamic by nature; containers spin up and down, workloads shift across environments, and metrics change by the second.
The result? An avalanche of alerts, false positives, and missed anomalies.
This is where AI monitoring tools for cloud provide a smarter, scalable approach.
What Are AI-Powered Monitoring Tools?
AI-powered monitoring tools; also known as AIOps tools (Artificial Intelligence for IT Operations); use machine learning, pattern recognition, and big data analytics to:
- Analyze large volumes of performance metrics, logs, and traces
- Automatically detect anomalies without predefined thresholds
- Correlate events across systems to pinpoint root causes
- Predict incidents before they impact users
- Recommend or automate remediation actions
This intelligent approach is critical for modern cloud observability, especially in environments that include multi-cloud, hybrid cloud, or microservices architectures.
Cloud Sovereignty Considerations
As organizations adopt multi-cloud and hybrid strategies, cloud sovereignty becomes a critical concern—especially for enterprises operating in regulated industries or across multiple jurisdictions. Ensuring that data remains compliant with local laws, residency requirements, and governance standards is essential for secure, scalable deployments. While AI-powered monitoring brings operational intelligence, it must be implemented within a framework that respects sovereignty mandates. To dive deeper into how to achieve compliance without sacrificing agility, explore our guide on achieving cloud sovereignty for secure deployments.
Key Benefits of AI in Cloud Infrastructure Monitoring
Let’s break down the most significant advantages that AI in cloud infrastructure brings to both technical and business teams.
1. Noise Reduction and Alert Precision
One of the major pain points of traditional tools is alert fatigue. AI-powered systems analyze the context of metrics and logs, grouping related alerts and suppressing noise. This helps operations teams focus only on what truly matters.
2. Faster Root Cause Analysis
With AI correlating data from across your tech stack; compute, storage, databases, and third-party services; you get a clearer picture of what’s really causing downtime or performance issues. This saves hours of manual log-diving and guesswork.
3. Proactive Issue Resolution
Machine learning monitoring tools can forecast performance degradation based on historical data. That means you can fix problems before they escalate, reducing mean time to resolution (MTTR) and improving uptime.
4. Cost Optimization
By analyzing usage patterns and resource allocation, AI can identify overprovisioned services or idle assets. This enables smarter spending and helps business owners trim unnecessary cloud costs.
5. Enhanced Developer Productivity
Developers can offload much of the heavy lifting in monitoring and debugging to intelligent systems, allowing them to focus more on building features rather than putting out fires.
Core Capabilities to Look for in Intelligent Monitoring Tools
When evaluating AI monitoring tools for cloud, here are some features you’ll want to prioritize:
Real-Time Anomaly Detection
Tools should not only alert you but also provide context—what changed, where it happened, and how it compares to historical patterns.
Log and Metric Correlation
AI that can correlate logs, traces, and metrics across services gives you holistic observability.
Predictive Analytics
Look for tools that go beyond reactive alerts and offer forecasts on capacity, performance trends, or possible failures.
Automated Remediation
Some AIOps tools can trigger auto-healing scripts or workflows in response to issues, further reducing downtime.
Multi-Cloud and Hybrid Support
If you operate across AWS, Devops Azure, Google Cloud, or on-prem, ensure your monitoring solution spans all environments seamlessly.
Popular AIOps and AI Monitoring Tools for Cloud
Here are some top players in the space that combine observability with AI:
Datadog AIOps
Datadog’s machine learning features help reduce alert fatigue and automatically surface relevant insights from infrastructure and application telemetry. Great for cloud-native and serverless environments.
Dynatrace
Dynatrace uses its Davis AI engine to automatically map dependencies, detect anomalies, and provide root cause analysis. It’s ideal for large-scale enterprise cloud deployments.
New Relic AI
New Relic One integrates AI/ML into its full-stack observability platform, offering anomaly detection, incident correlation, and intelligent alerting.
Splunk Observability Cloud
Combining logs, metrics, and traces with AI/ML capabilities, Splunk helps organizations predict and prevent incidents in complex cloud environments.
Moogsoft
A specialist in AIOps, Moogsoft uses AI to detect anomalies and automate incident resolution across hybrid and multi-cloud infrastructure.
Each of these tools has strengths, so the best fit depends on your architecture, team size, and specific use cases.
Business Use Cases: How AI Monitoring Translates to Value
E-commerce Platforms
During flash sales or high-traffic periods, AI tools can proactively identify scaling issues or bottlenecks before customers hit broken pages or slow checkout flows.
Fintech Applications
Monitoring high volumes of transactions requires precision. AI-based tools can detect subtle anomalies in performance or behavior that may signal deeper infrastructure or security issues.
SaaS Products
With multiple microservices and redefining CI/CD Workflows in play, AI observability ensures faster release cycles without compromising performance or reliability.
Media Streaming Services
Predictive monitoring ensures content delivery remains seamless, even during high usage times, by proactively allocating resources.
Challenges and Considerations Before Adopting AI Monitoring
While the benefits are substantial, transitioning to AI-powered observability comes with some considerations:
Data Volume and Privacy
AI tools require access to large datasets. You’ll need to ensure compliance with data security and privacy regulations.
Initial Learning Curve
AI models may need time to learn your environment’s “normal” behavior. During this phase, false positives can still occur.
Tool Sprawl
Many teams already use multiple DevOps tools. Adding yet another can create integration headaches if not planned carefully.
Cost Implications
AI monitoring platforms can come with premium pricing. But the ROI in terms of reduced downtime, saved developer hours, and improved user experience often makes up for it.
The Future: AI + Observability = Autonomous Infrastructure
The ultimate goal of integrating cloud observability AI tools is not just monitoring but autonomous operations.
Imagine an environment where:
- Incidents are predicted and prevented before they reach users
- Infrastructure self-heals using pre-trained workflows
- Developers are automatically notified of critical changes in code or performance
- Business decisions are driven by real-time insights from infrastructure health
That’s the promise of intelligent monitoring driven by AI and machine learning. And while we’re not fully there yet, leading-edge enterprises are already moving in that direction.
Final Thoughts: Smart Monitoring is Now a Business Imperative
Modern cloud infrastructure is too fast-moving and complex to manage with legacy monitoring systems. For businesses aiming to stay competitive, the adoption of AI monitoring tools for cloud is no longer optional; it’s essential.
Whether you’re a business leader looking to reduce costs and downtime or a developer aiming for faster incident resolution, AI in cloud infrastructure provides a scalable, intelligent way forward.
From anomaly detection to predictive analytics and autonomous remediation, AIOps tools bring the clarity, speed, and efficiency your cloud operations need in 2025 and beyond.
Ready to Bring AI Intelligence to Your Cloud Stack?
Looking to implement machine learning monitoring tools or exploring the best AIOps platforms for your business? Start with a pilot, evaluate based on your stack, and scale as your needs grow. The earlier you adopt intelligent monitoring, the better your position to deliver high-performing, resilient cloud services.
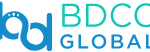
BDCC
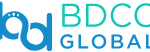
Latest posts by BDCC (see all)
- AI-Powered Monitoring Tools for Cloud Infrastructure - May 7, 2025
- DevOps Maturity Models: Where Does Your Organization Stand? - May 2, 2025
- The Role of Azure in Accelerating AI Adoption Across Industries - April 29, 2025